AI-Driven Customer Feedback Analysis: Optimizing Processes and Customer Service
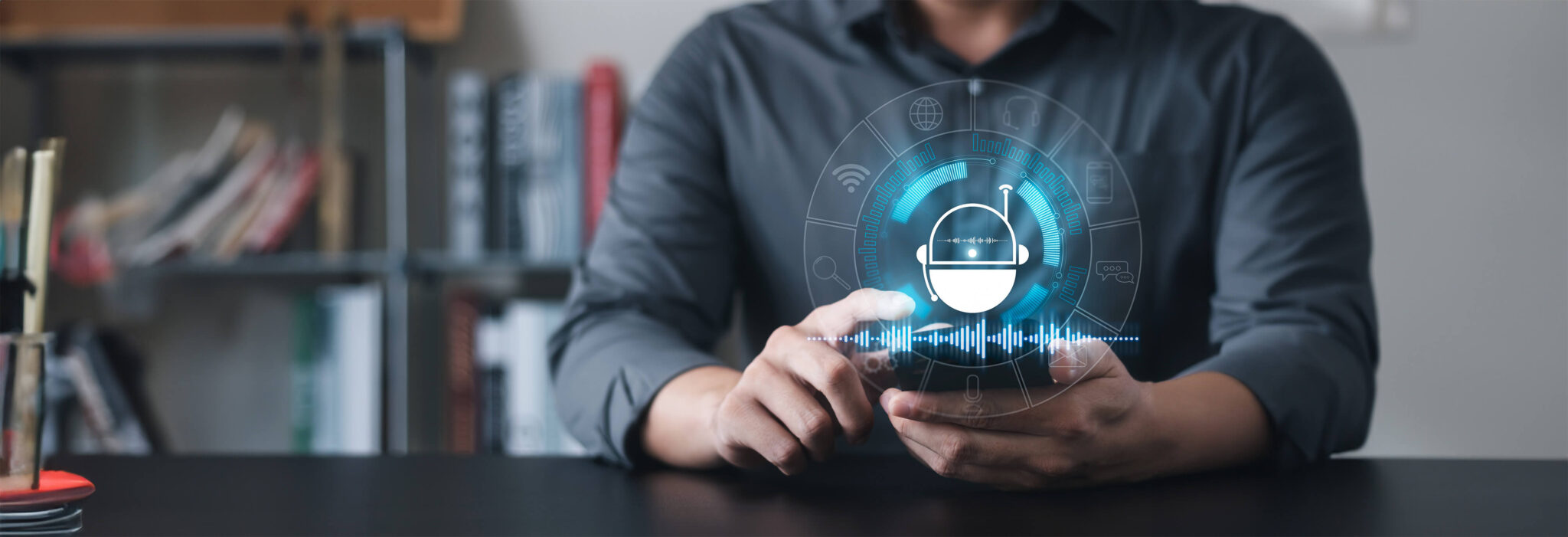
Overview
Acrontum developed an AI-powered system to automate the categorization of user feedback for a German automobile manufacturer. Previously, the feedback was manually processed in spreadsheets. Acrontum’s solution automatically collects, categorizes, and sorts the feedback, routes it appropriately, and integrates the data into PowerBI. This increased efficiency, reduced team size, and enabled faster responses and informed management decisions.
Client need
The client needed a solution to automate the categorization of user feedback to quickly generate statistics for internal management and identify issues early. The goal was to enhance the efficiency of feedback processing and ensure timely communication of key insights.
Highlight
The system processes 1,000 user comments daily across five markets, reducing the personnel required for feedback management from six to two team members.
Solution built
Acrontum developed an AI-based system that automatically collects, categorizes, and sorts user feedback by sentiment and topic. This enabled efficient routing of comments to the relevant staff and provided response suggestions. Additionally, the collected data was integrated into PowerBI for further analysis and reporting.
Services Used
Requirements Elicitation, Solution Conceptualization, Business- and Process Analysis, Wireframing and Testing, Data Analysis, Identification of AI-Algorithm
Client Situation
Approach
Outcome
Client Situation
The client received large volumes of user feedback through the Google PlayStore, Apple AppStore, and directly from the app, which were manually categorized in spreadsheets. This resource-intensive method caused delays in evaluating and responding to feedback. Manual categorization hindered the rapid creation of statistics for internal management and early identification of issues.
Approach
Acrontum analyzed the client’s existing feedback messages and categorization methods. Together, they defined the key criteria for the AI system. The acrontum team then evaluated various Large Language Models (LLMs) to select the most suitable model for the project’s requirements. After determining these requirements, the acrontum team developed an AI system that automatically collects feedback, categorizes it by sentiment and topic, and routes the comments to the relevant staff. The collected data was integrated into PowerBI for further analysis and reporting.
Outcome
The implementation of the customized AI system significantly increased the efficiency of processing user feedback. The system automatically categorized 1,000 comments daily, enabling the client to respond to feedback more quickly. The number of team members required to manage the feedback was reduced from six to two.
More of our work
- Cloud Infrastructure
Cloud Cost Dashboard Integration: Managing Expenses and Decision Making
A global automotive manufacturer needed a central solution to efficiently consolidate cost data from AWS and Azure. Acrontum developed a dashboard that integrated the cloud costs of both providers, offered extensive filtering options, and enabled efficient permissions management. This solution significantly improved the overview of cloud expenses and facilitated informed decision-making.
Concepting, development, implementation
- Business Process Optimisation
Integration Plan: Strategy Development for Cross-Market App Rollout
To implement a new vehicle information app in eight different markets with varying system landscapes, a globally active corporation needed a comprehensive integration plan. Acrontum developed precise architecture diagrams that depicted the integration options for each market, enabling informed decision-making and unified stakeholder alignment.
Concepting, development, implementation
- MVP Development
Operational Financial Planning App: Simplifying Invoicing and Improving Efficiency
Acrontum developed a financial planning app that automated and simplified the complex invoice approval process for a corporate department. Within three months, an MVP was delivered, automating key tasks, integrating with existing systems, and improving efficiency and security. This facilitated the review and booking of invoices from numerous suppliers against the available budget.
Concepting, development, implementation
Tagged case study